data science in photonics and medicine
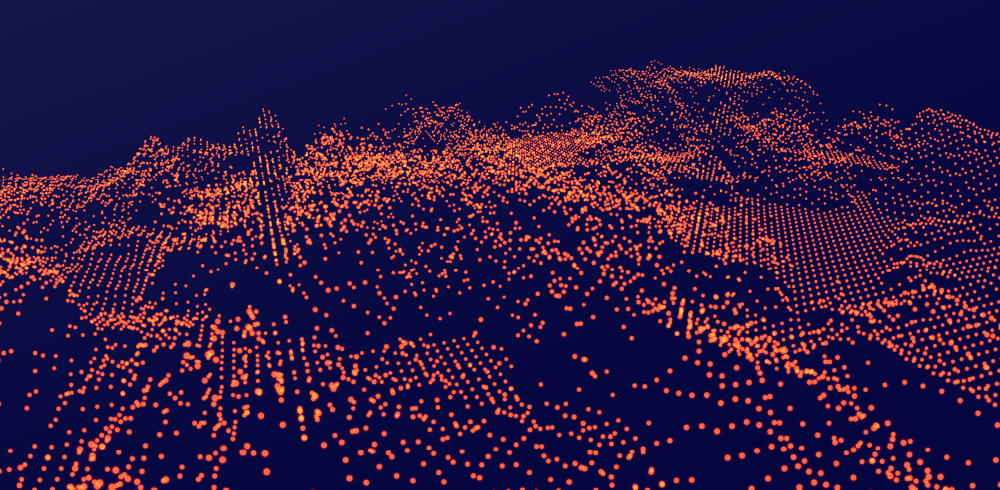
Research group of Dr. Kosmas Kepesidis
We explore the extent to which medical information acquired from photonic data can be utilized in medical diagnostics, personalized health monitoring, and life sciences. Specifically, we investigate relevant procedures for experimental and study design as well as data preprocessing. We combine these procedures with machine learning methods and ideas from medical statistics in appropriate data-science pipelines. The resulting pipelines are implemented using open-source software and directly tested on suitable clinical studies.
In addition, we investigate fundamental problems in medical decision-making from both a theoretical and data-driven point of view. Using ideas and tools from information theory, decision theory, as well as statistical physics, we aim for the quantification of medically relevant information carried by different types of health data sets. Furthermore, we seek to assess their utility for healthcare, and cross-compare their efficiency in precisely defining the health status of an individual.
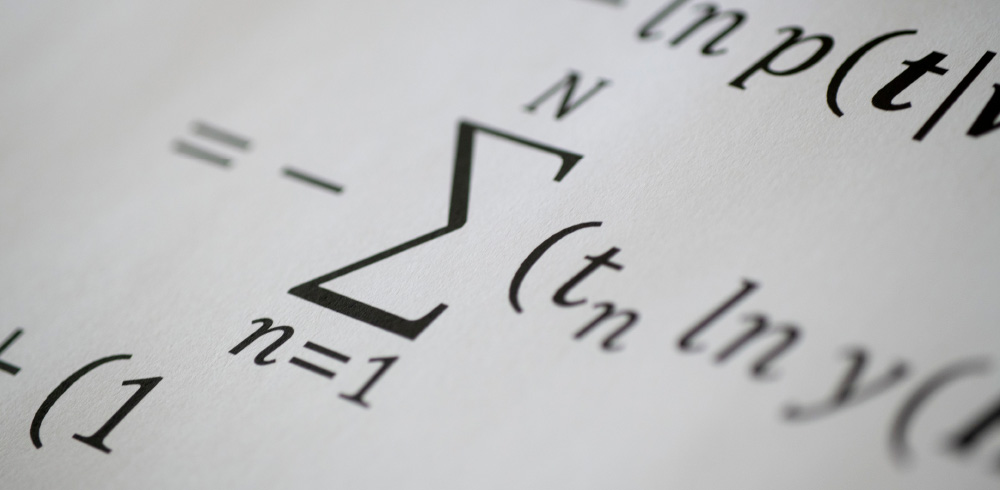
machine learning and domain adaptation
Based on case-control studies and infrared measurements of human blood, we train predictive models using classical machine learning methods that could aid disease diagnostics and screening. Additionally, we utilize longitudinal studies for the development of an infrared-based personalized health-monitoring system. Such systems, based on machine learning, often face major challenges when applied in practice, since their conditions of development differ from the conditions during clinical application. We try to overcome these challenges using ideas and methods from the fields of domain adaptation, transfer learning, and active machine learning.
medical statistics
Several investigations have shown the potential of applying molecular fingerprinting by vibrational spectroscopy combined with machine learning on medical problems, promising the development of new diagnostic or screening tests. All these works concentrate on the overall performance of such candidate medical tests. Only very limited research has been performed to assess the impact of factors beyond disease status that can affect the test result. By investigating such confounding factors using rigorous statistical evaluations based on theory developed in the field of medical statistics, we assess the potential and limitations of the proposed medical tests.
information-theoretic approach to disease monitoring
We aim to answer fundamental questions relevant to medical decision-making. Using ideas from information theory, we strive to assess the characteristics a medical dataset should possess to allow for the precise assessment of wellness and indicate wellness-to-disease transitions at an early stage, while being regularly acquirable at affordable cost.
artificial intelligence (AI) for in-silico clinical studies
The AI algorithms of the class of generative models (GMs) are designed to generate artificial but realistic data based on large sets of real observations. We experiment with GMs to be used in so-called in-silico clinical studies, which are virtual clinical trials conducted using a computer simulation. Such studies offer high potential to accelerate the development of new drugs, medical devices, and tests while significantly cutting R&D costs.
data engineering
We work towards best practices for designing and building systems to collect, store, and analyze scientific data at scale. This involves appropriate database design and the development of unified domain-specific scientific software packages for data analysis and processing.